Our Committees
OGC’s Committees are Central to our Success
OGC members can take part in a variety of committees that are both staff and member driven. They actively shape our work, from setting OGC’s strategic direction to steering our standards’ adoption.
Strategic Member Advisory Committee
The Strategic Member Advisory Committee (SMAC). The SMAC provides a forum for Strategic members to guide and influence OGC’s planning and support our operations.
Planning Committee
The Planning Committee (PC). OGC Strategic and Principal members can take part in the PC, which approves Technical Committee recommendations for the adoption and release of OGC standards. It also recommends and votes on appointments to OGC’s Board of Directors.
Technical Committee
The Technical Committee (TC). The main work on OGC Standards is led by the TC; it coordinates their development, modification and adoption. Sub-groups and sub-committees support the TC’s work. It is made up of all members of the OGC with voting privileges available to Voting members and above.
Additionally, the OGC Architecture Board (OAB) maintains consistency of the OGC Standards Baseline and evaluates candidate standards for their compliance with the OGC Modular Specification. It also evaluates current technology issues and trends. The OAB identifies gaps to be addressed by OGC members and approves all OGC Collaborative Solution & Innovation Program Interoperability Experiments. Membership of the OAB is by election.
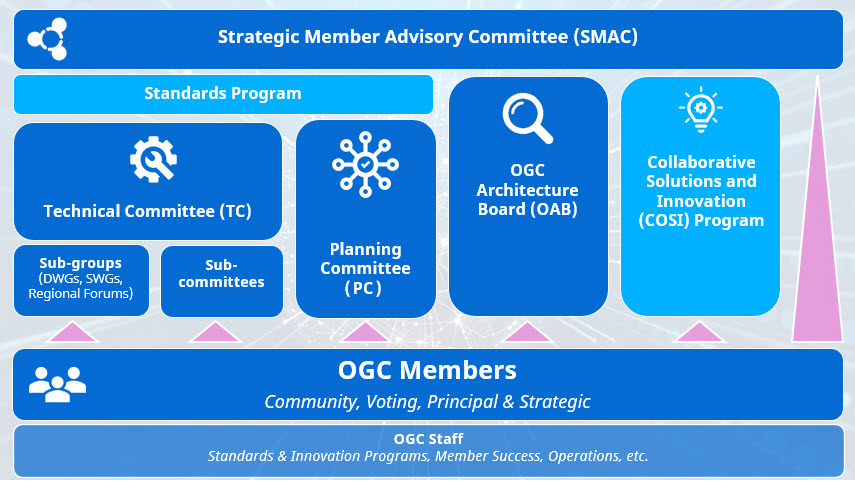